Car accessory recommendation
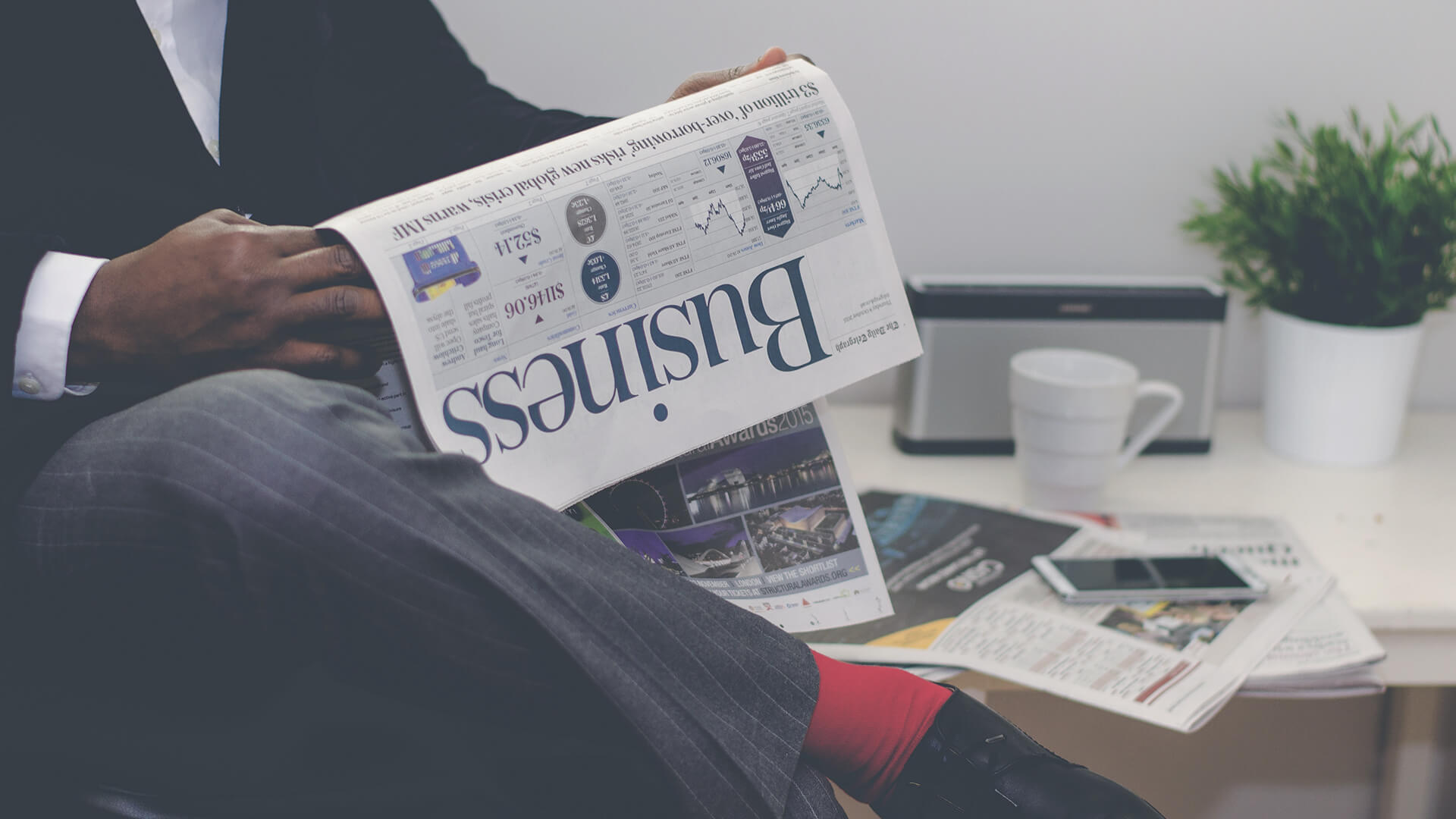
Challenge
AutoIntel is a New Jersey based startup that offers a data science platform to leading automotive OEMs. Their platform enables automotive companies to make use of online customer behavior, increase customer engagement and drive sales.
AutoIntel’s dataset, consisting of 34 million users interacting with 1600 different car models and options yielded around 8 billion positive and negative interactions. AutoIntel wanted to explore a number of research questions in the context of recommendation systems, such as what kind of recommender algorithm is most adequate to the data; does the inclusion of user or item metadata improve the performance of the recommender or how the different car options affect the model.
Our team developed a number of content-based filtering, collaborative filtering algorithms and other machine learning assets, such as cooccurrence features, text embeddings and performed feature importance analysis.
Results
F1 (content-base filtering): | +333% (over baseline) |
Precision at 3 (collaborative filtering): | +81% (over baseline) |
Recall at 3 (collaborative filtering): | +87% (over baseline) |
Explored the contributions of user metadata, item metadata and car accessories in the recommender models. |
The team helped us explore various different models and provided a great basis for decision making.
Julian Offermann
CEO of AutoIntel, Inc.
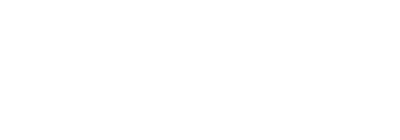